Chemometrics is a robust discipline, leveraging sophisticated tools and methodologies to extract critical insights from intricate data. It delves into a spectrum of applications and fundamental principles, emphasizing its pivotal position in both data processing and analysis. With discussions ranging from model-based scrutiny to handling multidimensional structures, and bridging chemometrics with diverse sectors.
Introduction to Chemometrics in Modern Analytics
In the world of cutting-edge analytics, chemometrics emerges as a vital instrument. It’s key for sifting through and making sense of complex data. This field uses high-level math and stats to draw important insights from convoluted information. We’ll dive into chemometrics here, underlining its essential role in today’s data analysis.
The Role of Chemometrics in Data Processing
Chemometrics tackles the cryptic data we see in many sectors. Its mission is clear: to craft methods that effectively digest large, intricate data. Employing sophisticated analyses, it detects patterns, uncovers connections, and pulls out the info we need from heaps of data.
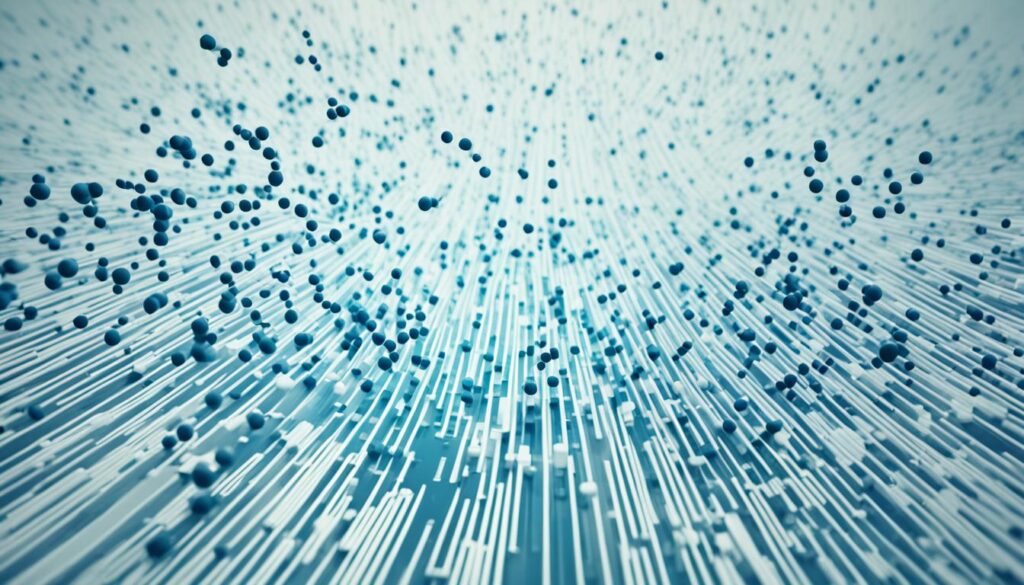
This approach lets us look at data in new ways, understanding complex systems better. It’s a game-changer for fields like pharmaceuticals, keeping an eye on the environment, food checks, and material studies. By using chemometrics, companies can smarten up, streamline, and foster new ideas.
Historical Context and the Evolution of Chemometric Techniques
Getting the full picture of chemometrics means knowing where it comes from. In the mid-20th century, a team of statisticians and chemists laid down the groundwork. They needed ways to make sense of complicated chemical data.
Since then, with tech progress and data complexity soaring in science, so have chemometric methods. What was once basic pattern spotting has blossomed into complex machine learning. Nowadays, chemometrics stands as a key player in the analytics field.
Decade | Chemometrics Milestones |
---|---|
1950s | Introduction of principal component analysis (PCA) by Harold Hotelling |
1960s | Development of discriminant analysis and partial least squares (PLS) |
1970s | Advancements in calibration and regression models |
1980s | Introduction of artificial neural networks and genetic algorithms |
1990s | Emergence of chemometrics software and widespread applications |
2000s | Integration of chemometrics with big data analytics and machine learning |
The table above showcases the major steps in chemometrics’ journey. Each milestone marks a vital shift, pushing the field forward and solidifying its place in analytics today.
Core Principles of Chemometric Analysis
To be effective in using chemometric analysis in research or industry, understanding its core principles is essential. We delve into the basic concepts and techniques in chemometrics. This includes data preprocessing, multivariate analysis, and model building.
Data Preprocessing
Data preprocessing is critical. It cleans, transforms, and normalizes data for reliable outcomes. This step removes outliers, manages missing data, and decreases noise to enhance data quality.
Multivariate Analysis
Multivariate analysis tackles the examination of multiple variables simultaneously. It identifies patterns, trends, and correlations between these variables, providing a deep insight into complex data sets.
Model Building
Model building focuses on creating mathematical models to forecast and elucidate data relationships. It entails choosing suitable algorithms, teaching models with labeled data, and confirming their efficacy.
Applications
These core principles underpin a range of chemometric analysis applications. These include enhancing quality control in manufacturing, predicting chemical properties, classifying samples, and improving experimental design.
Applications | Description |
---|---|
Quality Control | Ensuring consistent product quality through the identification of deviations in the manufacturing process. |
Prediction of Chemical Properties | Foreseeing chemical properties by analyzing spectral or chromatographic data. |
Classification | Sorting samples into different categories based on their chemical makeup. |
Experimental Design | Finding the best parameters for experiments to achieve the desired outcomes cost-effectively and eco-friendly. |
With a grasp of these foundational principles and applications, individuals can effectively use chemometric analysis in their projects. This knowledge is vital for various sectors, including research, development, and decision-making.
Enhancing Data Interpretation with Model-Based Analysis
Model-based analysis is a key aspect of chemometrics, elevating our ability to interpret data. It employs intricate statistical models to reveal profound insights from intricate chemical datasets. The discourse will delineate the superiority of model-based over model-free evaluation strategies. We’ll also review Edmund R. Malinowski’s pivotal work in demonstrating the power of model-based analysis.
Understanding Model-Free vs. Model-Based Evaluation
Essential in analyzing chemical data are both model-free and model-based approaches. Model-free methods do not assume statistical models, relying instead on direct observations. This approach features simplicity and flexibility, although it might compromise accuracy with intricate data.
Contrastingly, model-based assessment formulates statistical models to elucidate variable relationships. These models, rooted in math, unveil complex patterns and correlations. They empower accurate predictions and insights into chemical processes.
Model-based strategies offer numerous perks. They allow for the integration of prior knowledge about the system, enhancing prediction accuracy. These methodologies also facilitate hypothesis testing and allow for uncertainty to be quantified.
Case Studies: Malinowski’s Contributions to Chemometrics
Edmund R. Malinowski has left a profound mark in bringing model-based analysis to chemometrics. His work underscores the necessity of statistical models in gleaning insights from chemical data.
He is notably credited with devising PLS regression, a method that excels in predicting chemical features. PLS empowers the construction of models that can parse intricate relationships between variables, enriching data interpretation.
Malinowski’s expertise extends to experimental design, where he aids in the judicious selection of measurements and samples. This approach ensures both the efficiency of resource utilization and maximizes information gain.
His case studies highlight the application of modeling in areas like pharmaceuticals and environmental studies. They underscore the practical impact of model-based analysis in tackling complex, real-world challenges in chemistry.
Model-based analysis stands as an invaluable instrument in modern chemometrics. Contributions from luminaries such as Edmund R. Malinowski shape the field, enabling us to probe deeper into the workings of chemical systems.
Advancements in Multidimensional Separations
Chemometrics has seen great strides in the realm of multidimensional separations. It’s a state-of-the-art method allowing in-depth analysis of intricate samples. Using more than one method at once, researchers can go beyond single method constraints. This enables better resolution and sensitivity in their work.
The key to multidimensional separations lies in chemometric preprocessing. This includes steps like sample prep, extraction, and data conversion. These actions are critical in boosting the accuracy and precision of both qualitative and quantitative analyses. They clean up data, tackle biases, and improve signal clarity, yielding more dependable outcomes.
In this field, you’ll find two main strategies in play: targeted and nontargeted. The former zeroes in on spotting and measuring specific compounds. The latter takes a wider view, aiming to analyze all sample compounds without a set agenda. Each method is chosen depending on the study’s goals and fields of application.
For research focusing on particular compounds, targeted methods are the go-to. They use set targets and specific separation techniques to spot and measure compounds accurately. You’ll commonly see this in analyzing the environment, metabolomics, and pharmaceutical studies.
Conversely, nontargeted methods are ideal for those wishing to map out a sample’s complete chemical landscape without assumptions. This method is great for finding new compounds, recognizing intricate patterns, and understanding sample compositions more deeply. It’s frequently used in exploring food, monitoring the environment, and metabolomics.
Combining preprocessing with targeted and nontargeted strategies generates crucial insights in multidimensional separation. These insights carry broad applications – from analyzing complex biological systems to understanding environmental samples. They also aid in boosting industrial process quality checks.
Advantages of Multidimensional Separations | Targeted Approaches | Nontargeted Approaches |
---|---|---|
Enhanced resolution and sensitivity | Precise measurement of specific compounds | Exploration of the entire chemical space |
Improved accuracy and precision | Validated quantification of target compounds | Discovery of unknown compounds |
Comprehensive analysis of complex samples | Focused research objectives | Flexible and unbiased analysis |
The evolution of multidimensional separations, alongside preprocessing and distinct approaches, has profoundly enhanced chemometrics. This revolution is changing the way we analyze data. It arms researchers with powerful new techniques to unlock hidden truths in complex sample analysis. These breakthroughs are driving innovation across science.
Software and Tools for Chemometric Practitioners
Chemometric practitioners rely heavily on software and tools. These streamline their analysis and modeling tasks. The advanced features and functionalities of these tools enhance accuracy and efficiency.
SIMCA is a key software for such experts. It stands for Soft Independent Modeling of Class Analogies. This software empowers users to preprocess, build models, and validate them effortlessly.
It also provides visualization and statistical tools for deep analysis. It’s critical for interpreting complex data sets.
The Unscrambler is another essential in chemometrics. Known for its user-friendly interface, it covers a wide array of analytical tools. It’s perfect for tasks like exploratory analysis, classification, regression, and process optimization.
This software includes multiple predictive modeling and statistical methods. These enable practitioners to identify hidden patterns and derive meaningful insights.
For spectroscopy professionals, Grams AI is a game-changer. It merges chemometrics with AI, allowing for the analysis of spectral data using ML algorithms. This makes creating calibration models and performing predictive modeling much more straightforward.
As for open-source options, R is highly favored. It’s a statistical computing programming language with a vast variety of packages. These are tailored for chemometrics, enabling advanced analysis, visualization, and modeling.
R’s flexibility and breadth of features make it ideal for crafting customized data solutions. It’s a powerful ally for those with specific data needs.
Moreover, the field thrives on resources like books, tutorials, and forums. Sites such as Chemometrics World and Chemometrics Toolbox offer a wealth of information and discussion. This facilitates keeping up with the latest trends and methodologies, promoting collaboration.
With the right software and resources, chemometric experts can boost their analysis capabilities. They can make significant findings in their field. The growth in technology offers more sophisticated tools. These empower practitioners to explore new horizons in data analysis and discovery.
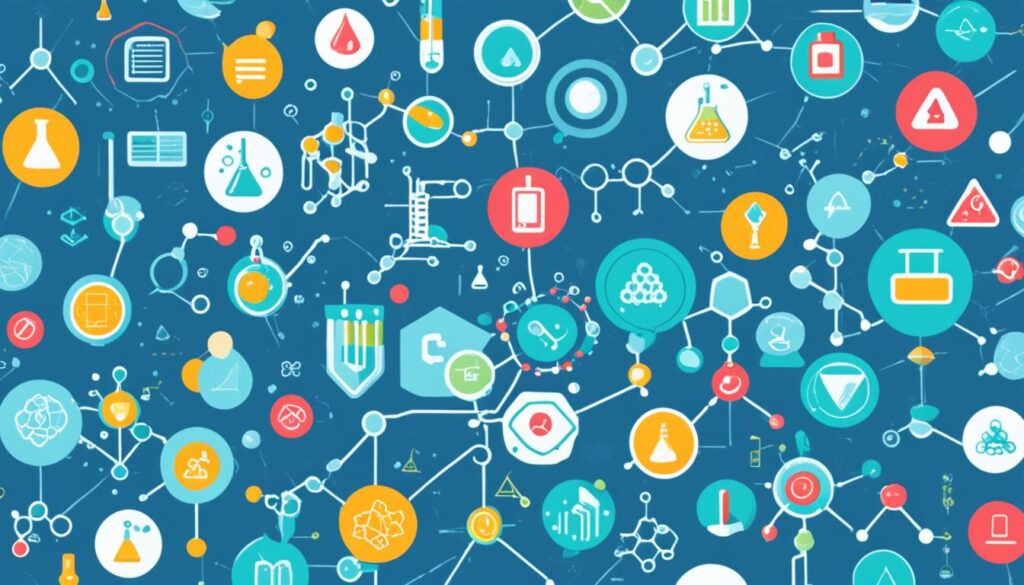
Application of Chemometrics in Spectrometry
Chemometric Strategies for Spectral Data Analysis
Spectrometry heavily depends on chemometrics for meticulous data examination. These methods employ diverse strategies to decode spectral data, bringing forth insights and meanings. One pivotal step is preprocessing the data, which tackles noise and baseline issues in the spectra. This enhancement improves the data’s precision and dependability.
Post preprocessing, the data engages in multivariate analysis, a key chemometric technique. Multivariate analysis explores the intricate relationships within spectral variables. This process aids in the identification and understanding of varied components. It illuminates hidden patterns and associations that evade simpler methods.
Chemometric analysis incorporates pattern recognition as well. This aspect aims to spot specific spectra linked with known substances or classes. It supports the classification, differentiation, and identification of mysterious samples by their spectral patterns.
Calibration Models and their Significance
Calibration models play a pivotal role in spectrometry’s chemometric arsenal. They correlate known spectral data with properties or concentrations, forming predictive models. These models extrapolate this relationship to understand unknown samples, enabling precise analysis.
Their value lies in enabling accurate and numerical analysis. Calibration models link spectral data to concrete properties, allowing for direct quantification of analytes. They also serve as measures for the performance and dependability of spectrometric tools.
In summary, chemometrics enriches spectrometry by extracting rich meaning from spectral data. With preprocessing, multivariate analysis, and pattern recognition, it’s possible to unveil the secrets of complex datasets. Calibration models then fortify the quantitative analysis and trustworthiness of spectrometry’s findings.
Chemometrics in Biotechnology and Pharmaceutical Industries
Chemometrics significantly impacts the biotechnology and pharmaceutical sectors. It uses advanced data analysis to spur innovation and refine operations. This exploration will delve into the integration of artificial intelligence (AI) and process analytical technology (PAT) in life science research. We’ll also cover their joint efforts with chemometrics. Additionally, we’ll review successful case studies. These studies will show how chemometric solutions address practical problems.
Deploying AI and PAT in Life Science Research
AI and PAT are essential in biotechnology and pharmaceuticals. They help in advancing research and development. Specifically, AI sorts through vast data, finding patterns and predicting outcomes. This aids in faster drug discovery, better process optimization, and stringent quality control.
PAT focuses on controlling manufacturing factors in real time. It allows for deep insights into production complexities. By adding chemometric insights, scientists ensure product quality consistency. They can also make smarter decisions to boost process efficiency.
The fusion of chemometrics, AI, and PAT opens up new avenues in drug development. It also enhances process optimization and quality assurance. This synergy is essential to push the biotechnology and pharmaceutical sectors forward.
Case Studies from Chemometric Solutions
We will present key case studies to illustrate how chemometric solutions benefit the sectors.
Case Study 1: A top pharmaceutical firm used chemometrics to understand its processes better. They optimized the formulation of a complex drug product. Applying advanced models and analysis, they pinpointed key process variables. This led to a more robust process control. The result was better product quality and lower costs.
Case Study 2: In biotechnology, chemometrics was vital for a predictive model. This model monitored growth characteristics of a specific bacteria strain. By merging chemometric insights with AI, growth outcomes were accurately predicted in real time. This allowed for early issue detection and proactive management for optimal growth conditions.
These studies highlight the impact of chemometrics in problem-solving and innovation. Through chemometric, AI, and PAT integration, industry professionals can streamline processes, improve quality, and expedite discoveries.
The Intersection of IoT and Chemometrics
The interaction between the Internet of Things (IoT) and chemometrics creates new paths for data analysis and integration. This integration allows for real-time oversight and control of industrial processes, blending the strengths of IoT and advanced chemometric analysis.
IoT devices contribute large volumes of data across sectors like manufacturing, farming, healthcare, and energy. Chemometric assessment allows for this data to be utilized, improving efficiency, quality control, and decision-making.
Real-time data collection from IoT sensors and devices is a primary advantage of uniting IoT with chemometrics. This allows for the continuous monitoring of vital variables, pinpointing any abnormalities or deviations promptly. With chemometric analysis, crucial insights are extracted, enhancing predictive capabilities and making way for actionable decisions.
By delving into IoT data with chemometrics, processes can be fine-tuned, cutting down on waste and upping resource use efficiency. This reveals how different variables interact and influence the process, helping decision-makers drive productivity and improve operations.
Yet, there are hurdles to overcome when merging IoT and chemometrics. Coping with the flood of IoT data poses a significant challenge. It demands proficient methods of data management to ensure data quality throughout the analytical journey.
Moreover, the compatibility of IoT devices and their data is another complex issue. For IoT data to be effectively used in chemometric analysis, compatibility across devices and data formats is necessary. Standardization of platforms and APIs plays a key role in tackling these issues, ensuring smooth data integration.
The progress in marrying IoT with chemometrics presents wide-ranging benefits across sectors. From refining manufacturing processes to advancing agriculture and health outcomes, this convergence fosters innovation. With sustained advancements in sensor tech, analytics, and machine learning, the potential of this field continues to grow.
Chemometrics
This section delves into key techniques and methodologies within chemometrics. It’s a robust field that blends various statistical and mathematical tools. This fusion is crucial for unpacking complex data, offering crucial insights for decision-making in areas such as chemistry, pharmaceuticals, and biotech.
Essential Techniques and Methodologies in Chemometrics
Chemometrics covers a broad spectrum of tools for data analysis and interpretation. Essential techniques within it are designed to handle complex data with finesse.
For instance, Principal Component Analysis (PCA) simplifies large data sets but keeps essential variations. Partial Least Squares (PLS) models complex relationships between different variables. Meanwhile, Cluster Analysis groups similar data to reveal patterns.
Other methods like Classification and Regression Trees (CART) are used for predictive modeling. Furthermore, Artificial Neural Networks (ANN) mimic the biological brain’s learning methods for intricate analysis.
All these techniques are essential in chemometrics. They help reveal insights, patterns, and accurate predictions from often intricate data sets.
Current Trends and Future Directions in the Field
Chemometrics is in a state of constant growth. It adapts to the latest advancements in data analysis, machine learning, and AI. Current trends and future directions reflect this evolution.
For example, there’s a drive towards integrating big data into the field. As data sets grow, chemometrics is harnessing tools like deep learning and cloud computing. Data fusion is also gaining traction, combining data sources for more robust models.
In addition, its application extends to real-time monitoring and control systems across industries. This leads to proactive decision-making and optimized processes. Techniques are also being utilized in omics sciences, exploring biological big data.
Advancements in spectral analysis refine our ability to quantify and identify compounds accurately. These developments underscore the ongoing growth and impact of chemometrics on various fields.
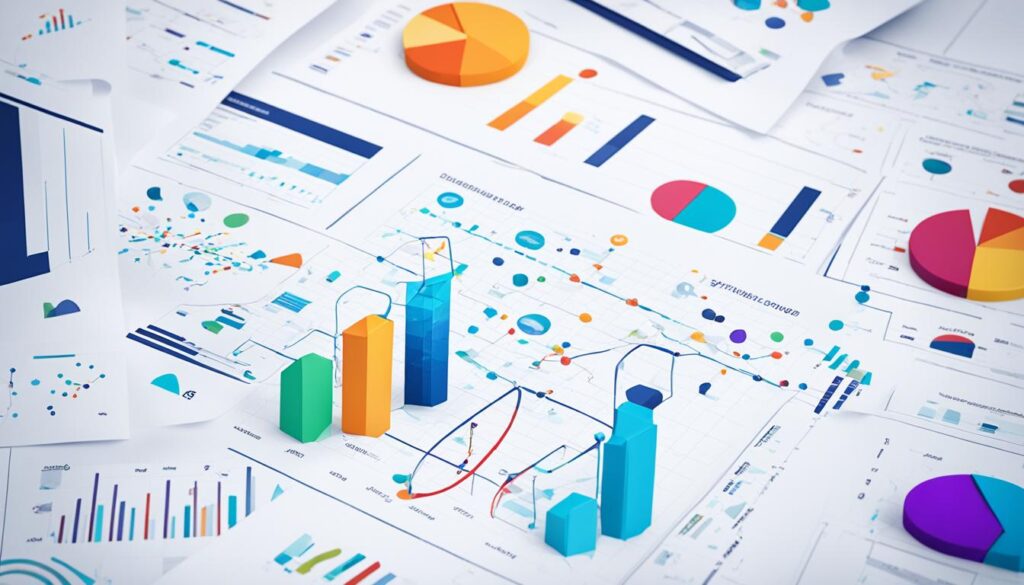
Optimizing Laboratory and Industrial Processes with Chemometrics
Chemometrics is vital for refining processes in labs and industries. It uses advanced data analysis. This lets businesses discover key insights. They then use this information to make smart choices. These choices boost efficiency, productivity, and maintain high quality.
Predictive Analytics and Quality Control Applications
Predictive analytics is key in chemometrics. It helps foresee and tackle issues early on. Companies can create models that predict outcomes. This proactive approach means problems are fixed before they impact quality.
Chemometrics merges data from different sources in real-time. It allows for swift monitoring. By identifying unexpected patterns, prompt actions can be taken. This decreases defect risks and improves process efficiency.
Process Simulation and Control through Chemometric Methods
Chemometrics equips businesses with tools for precise process control. They can assess and refine their processes. Through mathematical models, they simulate various scenarios. This provides insights and helps in making informed decisions for process enhancement.
Additionally, it supports real-time process adjustment. This ensures the best operating conditions are maintained. Liquidity, efficiency, and resource saving are promoted. With this, companies can operate with enhanced precision and reliability.
Chemometrics’ use in predictive analytics, quality assurance, and process management is critical. It is a key driver of success for modern businesses. By embracing data analysis and modeling, companies can stand out and excel in their field.
Conclusion
Chemometrics stands as a key player in the realm of data analysis, sparking a paradigm shift. It equips researchers and experts with sophisticated tools to navigate complex data with ease. The field’s impact spans widely, touching areas from biotech and pharma to spectrometry and IoT applications.
The role of chemometrics in processing data is crucial. Its principles allow researchers to draw out significant insights from intricate data. This approach has propelled developments in various areas, like more detailed separations and advanced analysis models.
The future for chemometrics is bright, driven by technological progress. We are likely to see enhanced methods through AI, process analytical technology, and IoT. This will pave the way for cutting-edge monitoring, predictive insights, and finer process controls.
To sum up, chemometrics is indispensable for understanding and innovating data across sectors. Its tools empower experts to glean valuable information from vast data sets. In doing so, it fuels progress and refinement in multiple industries.